Recently I was at at the Gitex AI Global Conference and I heard from an esteemed professor the concept of Data Winter. I was very curious about it and here’s an article to elaborate around it.
What is a Data Winter?
A Data Winter is period of dearth of interest and investment in sharing and making data available, along with lowered activity, usually induced by organizations not wanting to share their data. This may create major setbacks in data-driven innovation and research, similar to past “AI winters,” where funding and enthusiasm for AI research dwindled.
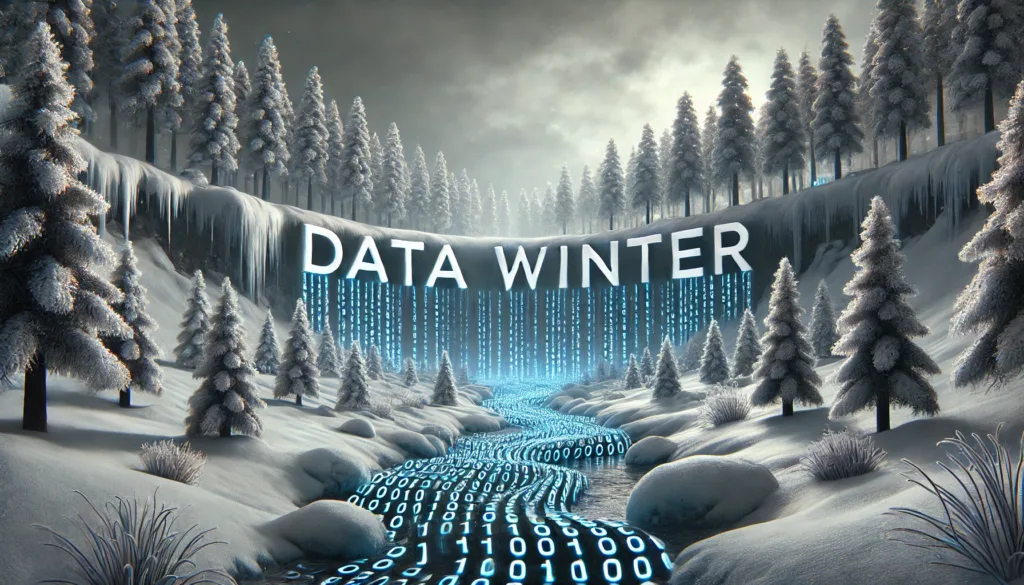
Causes of Data Winter
- Fear of Misuse: The organization is afraid to share the data because it feels that sharing data can lead to misuse or breach of privacy, thereby creating a culture of hoarding data. This fear is heightened by concerns about regulatory compliance and its associated legal implications.
- Regulatory Barriers: Generally, highly restrictive data protection regulations may lead to the general view that data sharing is a very risky operation. Organizations might interpret these regulations as prohibitive rather than frameworks that can actually facilitate responsible sharing.
- Siloed Data Practices: Most organizations are still working within internal silos where departments either compete for resources or simply do not trust one another enough to share information. Such isolation inhibits collaboration, innovation, and efficiency.
- Technological Challenges: The absence of different tools and technologies creates difficulty in sharing data effectively. Organizations might face interoperability issues where sharing data across multiple platforms or departments becomes difficult to tackle .
- Cultural Resistance: Lack of trust inside the organization builds up different kinds of fears related to data sharing. If the employees feel that data sharing can cost them their position or may create more conflicts, they will not like to collaborate .
Aftermath of Data Winter
The results of such a Data Winter are profound and world-altering:
- Stagnation of Innovation: reduced data sharing limits leveraging shared insight to drive innovation, hindering progress in fields that impact such pressing areas as health care, climate science, and social research.
- Inefficiencies: organizations that are unable or simply unwilling to share data may find themselves having to bear the brunt of operational inefficiencies, wasted resources, and inflated costs associated with maintaining underutilized data assets.
- Public Interest Concerns: all these restrictions to data access also impede research useful to society at large. According to some, this may be going toward the “digital dark age” where many insights remain locked up.
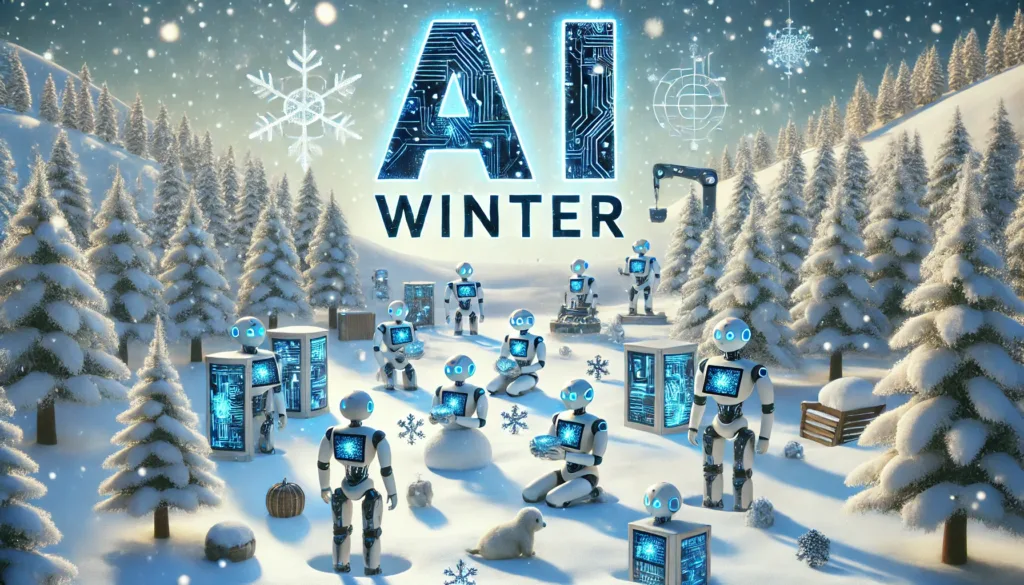
What’s an AI Winter, and How Does It Happen?
An AI Winter occurs when the hype surrounding AI is overtaken by skepticism. It may be caused by technical issues, over-promising, or even an economic downturn. In the past cycle, over-inflated expectations of expert systems-software programmed to think a lot like a human-blew up in disappointment during the 1980s. Since all enthusiasm plummeted, funding did too; hence, innovations slowed for almost a decade.
These winters tend to occur when there’s a mismatch between marketing narratives and real-world progress. Seeing the limitations of technologies nowadays, such as large language models generating false information, some are concerned we could be inching back toward another winter.
AI Winters came and went, but data presents different kinds of challenges. It’s not a question of tech burnout, but how we ensure data becomes a dependable backbone to unleash the AI potential. The onset might be sooner or later, but AI Data Winter will indeed test the very foundations of innovation-the going forward effectively depends upon how well the turbulence is weathered.
In light of such factors, a Data Winter caused by organizations being averse to data sharing is fraught with formidable challenges, and a cultural fears-regulatory frameworks-technological barriers approach is needed for a truly collaborative, innovative environment. This may trigger indeed a painful AI Winter affecting both generative and predictive artificial intelligence use case.
Leave a Reply
You must be logged in to post a comment.