AI hallucinations in large language models can lead to surprising and often misleading outputs. Imagine you’re asking your AI assistant about scientific concepts or historical facts, and it responds with confident, yet entirely fictitious explanations. These aren’t just simple mistakes; they represent a complex challenge known as “AI hallucination,” where the model generates plausible but incorrect or non-existent information. This occurs due to the AI’s training on vast, diverse datasets without truly understanding the content, leading to overconfidence in its responses. To navigate this, developers implement strategies like refining training data, enhancing model testing, and employing precise prompt engineering to ensure more accurate and reliable outputs.
What are AI Hallucinations?
You’ve probably encountered situations where AI like ChatGPT or Gemini outputs something bizarre or blatantly false. This phenomenon, known as “AI hallucination,” involves the AI generating information that isn’t just inaccurate but often confidently presented as fact. Essentially, these models sometimes “see” patterns or data that aren’t there, a bit like a mirage in a desert.
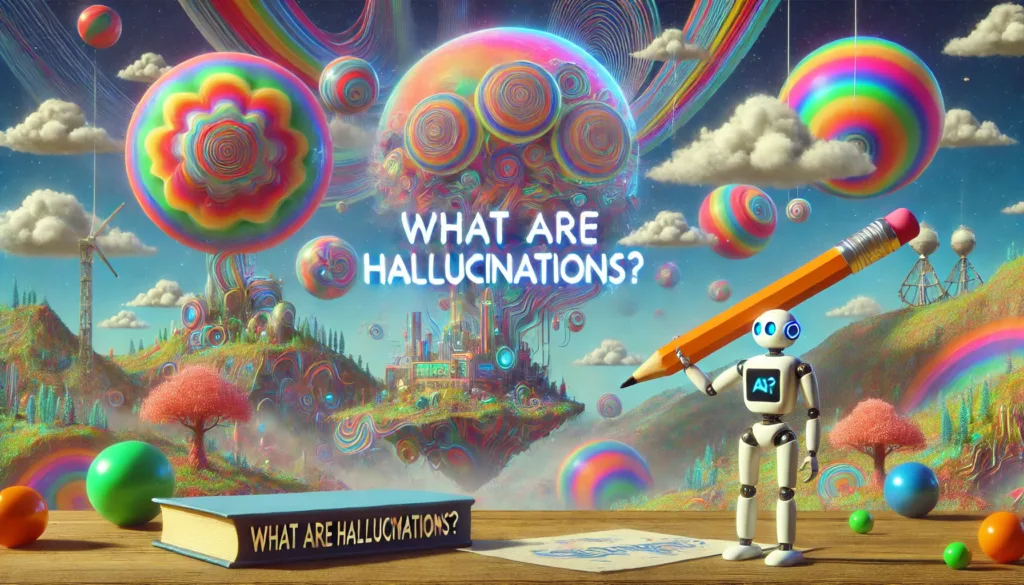
Why Do These Hallucinations Happen?
The roots of AI hallucinations can be traced back to several factors. First, there’s the training process, where models are often taught using techniques like maximum likelihood estimation. This method can inadvertently encourage models to replicate training data without genuine understanding, leading to errors when the AI encounters something it wasn’t specifically trained on. Additionally, when models like GPT-3 are pre-trained on vast amounts of text data, they may develop overconfidence in the ‘knowledge’ they’ve memorized, making them prone to asserting falsehoods with high confidence as the conversation or the text grows longer.
GPT-3 is heavily subject to Hallucinations while GPT-4 is much less prone to it, GPT-5 will hopefully have even less.
How Can We Minimize These Hallucinations?
Reducing AI hallucinations is not straightforward, but there are several strategies that developers use. One crucial approach is using high-quality, diverse, and well-balanced training data, which helps mitigate biases and provides a more comprehensive foundation of knowledge for the AI. Another effective method is prompt engineering, where the way questions are structured can significantly influence the accuracy of the AI’s responses. By specifying and narrowing down the context, you can often guide the AI to more accurate and relevant outputs. Continuous testing and refining of these models also play a critical role in catching and correcting hallucinations before they reach the user.
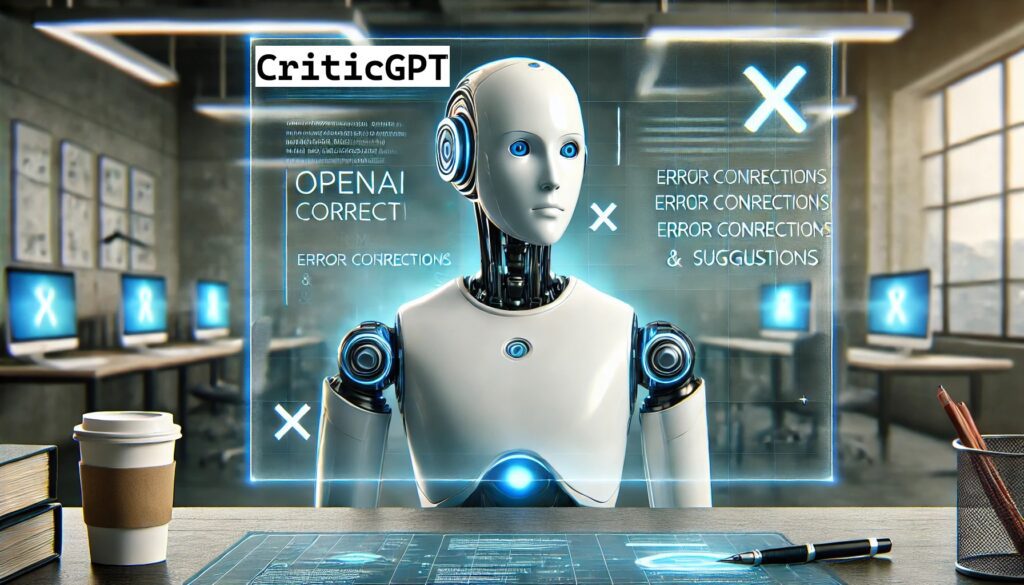
Is there any LLM that can help reducing hallucinations?
CriticGPT can be instrumental in reducing hallucinations in language models by applying an offline reinforcement learning approach. This method, used in task-oriented dialogues, involves fine-tuning a pre-trained language model like GPT-4 through what’s known as behavior cloning of critic-guided self-generated sentences. Essentially, CriticGPT learns from a curated set of sentences that have already been evaluated by the critic mechanism, allowing it to refine its output towards more accurate and contextually appropriate responses. This process helps to minimize deviations from human-like language by providing a feedback loop where the model’s outputs are continually assessed and corrected, leading to improved reliability and fewer hallucinations