Retrieval-Augmented Generation (RAG) is a fascinating technique that enhances the capabilities of language models by integrating them with external, reliable data sources. This method allows models like GPT to access up-to-date and relevant information beyond their initial training data, making them much more effective in generating responses that are accurate and contextually rich.
When you interact with a system equipped with RAG, your query triggers a two-part process. First, the system searches through a vast database, pulling information that best matches your question. This data, now transformed into a format the language model can understand, is then fed into the model. Leveraging both the retrieved data and its own training, the model crafts a response that not only addresses your query but does so with the most current and precise information available.
Practical Uses of Retrieval-Augmented Generation
The practical applications of RAG are extensive and varied. Businesses use RAG to power customer support chatbots, provide personalized recommendations, and enhance decision-making processes by quickly summarizing large volumes of text. For example, a financial analyst might use a RAG-enhanced system to pull the latest market data before it generates forecasts and reports, thereby ensuring that the information is both accurate and relevant.
Setting Up Your First RAG System
Getting started with RAG might seem daunting, but it’s quite achievable with the right tools. Platforms like NVIDIA’s NeMo provide a framework to build and customize your RAG systems. These platforms often come with sample applications, allowing you to see RAG in action and modify it according to your needs. The key is to integrate RAG with databases or knowledge bases that are tailored to your specific application, ensuring that the system has access to the most pertinent and up-to-date information.
Final Thoughts on RAG
Implementing RAG can transform how your applications interact with users by making them more informed and responsive. However, the quality of output heavily depends on the quality of the data retrieved and the efficiency of the language model. Regular updates and checks on the data sources are crucial to maintaining the effectiveness of a RAG system. While RAG systems are powerful, they require thoughtful integration and maintenance to fully harness their potential.
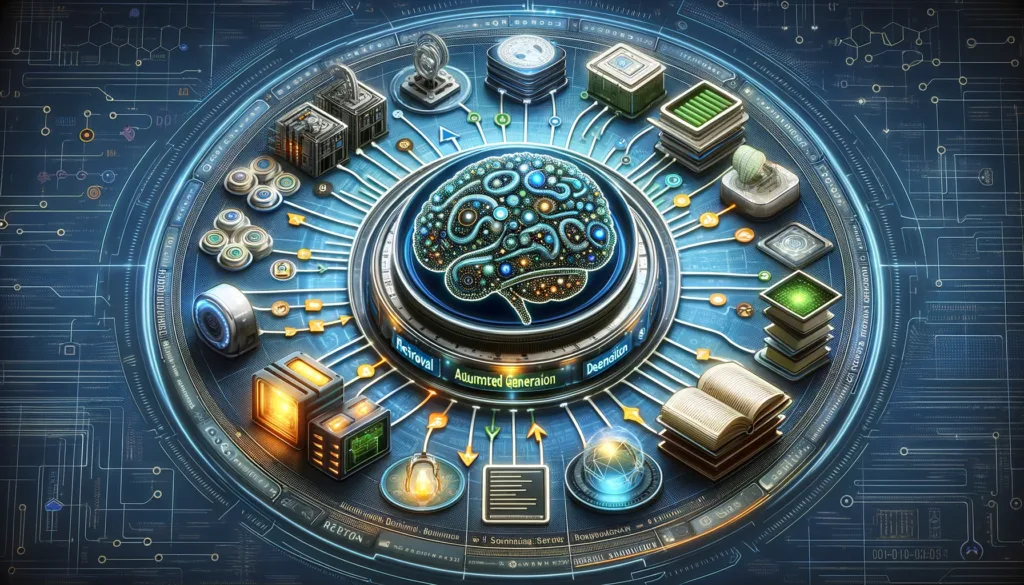
For those eager to explore more about retrieval-augmented generation and begin developing with this technology, understanding the basics and considering the scope of application in your own business or field of interest is a great starting point.