Are you trying to boost the reasoning capabilities of language models for complex tasks? Enter Chain of Thought (CoT) prompting, a method that significantly enhances the problem-solving abilities of large language models (LLMs) by guiding them through a series of logical steps before arriving at an answer.
Chain of Thought prompting is not just about getting the right answer; it’s about understanding how to get there. This technique breaks down a task into digestible, logical steps, allowing the model to handle each component one at a time. This method proves especially effective in tackling intricate problems that require detailed reasoning, such as mathematical calculations, commonsense queries, and even symbolic reasoning tasks. Researchers have found that models perform better when they generate intermediate steps that logically lead to the conclusion, rather than jumping directly to the answer.
How to Implement Chain of Thought Prompting
Implementing CoT prompting involves crafting your prompts to include a clear, step-by-step breakdown of how to approach the problem. Begin by outlining a problem in a way that naturally leads the model to consider each part of the task separately. For example, if the task involves complex arithmetic, guide the model through each calculation step before combining results for the final answer.
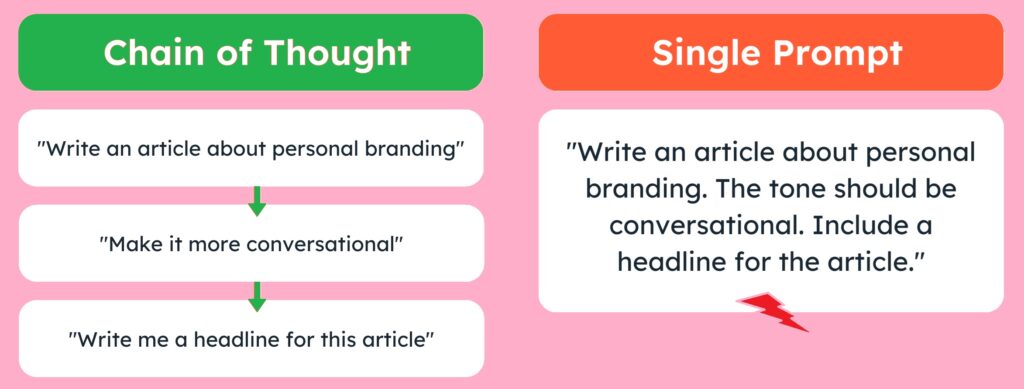
This approach is not only beneficial for accuracy but also for control and coherence in the responses generated by the model. By maintaining a structured chain of reasoning, the model preserves context better and provides responses that are not only correct but also contextually relevant and detailed.
CoT Benefits and Best Practices
One of the standout benefits of CoT prompting is its ability to significantly improve the performance of LLMs on a variety of benchmarks. This includes not just numerical tasks but also those requiring commonsense reasoning and understanding of complex scenarios. Moreover, CoT prompting enhances the model’s control over its outputs, reducing the risk of errors and irrelevant responses.
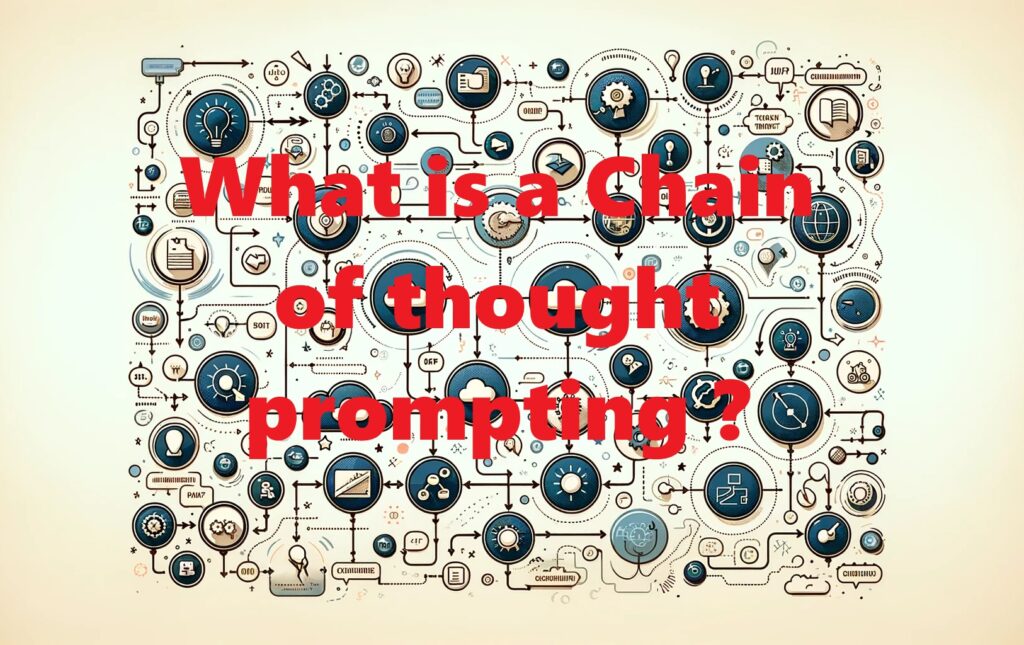
When you’re ready to try CoT prompting, remember to tailor your prompts carefully to match the specific requirements of your task. The prompts should be clear, concise, and structured to facilitate easy understanding and logical progression for the model.
By integrating Chain of Thought prompting into your generative ai workflow, you harness a powerful tool that not only boosts model performance but also deepens the model’s understanding of the tasks at hand, making it an invaluable technique for anyone working with advanced language models.